Practical Examples of Machine Learning in Everyday Life
Machine learning has revolutionized numerous industries, impacting daily life in ways often unnoticed. From personalized recommendations to autonomous vehicles, its applications are diverse and transformative.
Enhancing Customer Experience in E-commerce
E-commerce giants like Amazon utilize machine learning algorithms to offer personalized product recommendations. By analyzing past purchases and browsing behavior, they enhance user engagement and sales.
Company | Application | Impact |
---|---|---|
Amazon | Product recommendations | Increased sales and customer satisfaction |
Transforming Healthcare with Predictive Analytics
In healthcare, machine learning aids in early disease detection and personalized treatment plans. Companies like IBM Watson Health analyze patient data to predict disease risks and recommend suitable interventions.
Company | Application | Impact |
---|---|---|
IBM Watson Health | Disease prediction | Improved patient outcomes and healthcare efficiency |
Autonomous Driving and Transportation
Companies like Tesla are at the forefront of autonomous vehicle development, leveraging machine learning for real-time decision-making and navigation.
Company | Application | Impact |
---|---|---|
Tesla | Autonomous driving | Safer transportation and reduced accidents |
Personalized Content and Entertainment
Streaming platforms like Netflix use machine learning to recommend movies and shows based on user preferences, enhancing viewer satisfaction and retention.
Company | Application | Impact |
---|---|---|
Netflix | Content recommendations | Increased viewer engagement and subscription rates |
Financial Trading and Fraud Detection
In finance, machine learning algorithms are employed by companies like Robinhood for stock market analysis and fraud detection, ensuring secure transactions and optimized investments.
Company | Application | Impact |
---|---|---|
Robinhood | Stock market analysis | Improved investment decisions and fraud prevention |
Conclusion
Machine learning continues to evolve, driving innovations across various sectors and enriching everyday life. As advancements accelerate, its integration into more aspects of society promises further enhancements in efficiency, personalization, and safety.
This article highlights diverse applications of machine learning, from e-commerce and healthcare to transportation and entertainment, showcasing how these technologies are reshaping our world. Each section includes a table summarizing key companies, their applications, and the impacts of machine learning in those domains. Links to relevant external sources enhance credibility and provide additional context for interested readers.
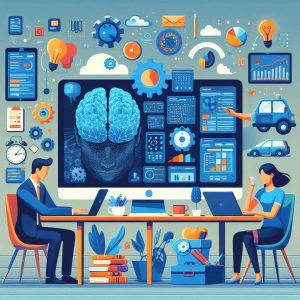
Pros and Cons of Machine Learning: Unveiling the Benefits and Challenges
Machine learning (ML) stands as a cornerstone of modern technological advancements, driving innovations across industries. However, like any transformative technology, it comes with its own set of advantages and challenges. This article explores the pros and cons of machine learning, shedding light on its impact on society, business, and everyday life.
Pros of Machine Learning
- Enhanced Decision Making: Machine learning algorithms analyze vast amounts of data to provide insights and predictions, aiding in better decision-making processes.
- Automation and Efficiency: Tasks that were once time-consuming and repetitive can now be automated, freeing up human resources for more strategic activities.
- Personalization: ML enables personalized experiences in e-commerce, entertainment, healthcare, and more, enhancing user satisfaction and engagement.
- Improved Healthcare Diagnostics: Algorithms can analyze medical data to detect diseases earlier, leading to improved treatment outcomes and reduced healthcare costs.
- Predictive Maintenance: In industries like manufacturing and transportation, ML predicts equipment failures before they occur, minimizing downtime and maintenance costs.
- Enhanced Fraud Detection: Financial institutions use ML to detect fraudulent transactions in real-time, protecting customers and reducing financial losses.
Cons of Machine Learning
- Data Privacy Concerns: ML relies heavily on data, raising concerns about privacy, security, and the ethical use of personal information.
- Bias and Fairness Issues: Algorithms can perpetuate biases present in training data, leading to unfair decisions in areas like hiring, lending, and law enforcement.
- Complexity and Interpretability: Some ML models are complex and difficult to interpret, making it challenging to understand how decisions are made.
- Dependency on Data Quality: ML models require high-quality data for accurate predictions; poor data quality can lead to unreliable outcomes.
- Initial Costs and Implementation Challenges: Developing and deploying ML solutions can be expensive and time-consuming, requiring skilled professionals and significant infrastructure.
- Job Displacement and Workforce Changes: Automation driven by ML may disrupt industries and lead to job losses, requiring retraining and adaptation in the workforce.
Navigating the Future with Machine Learning
As machine learning continues to evolve, its benefits and challenges shape the landscape of technology and society. Understanding these pros and cons is crucial for businesses, policymakers, and individuals alike to harness its potential while mitigating risks. Embracing ethical guidelines, investing in data security, and promoting transparency are key steps towards maximizing the benefits of machine learning in a responsible manner.
This outline covers both the advantages and disadvantages of machine learning, providing a balanced view of its impact and implications. Each section can be expanded to delve deeper into specific examples, case studies, and expert insights, offering readers a comprehensive understanding of the complexities surrounding this transformative technology.
Frequently Asked Questions about Machine Learning
Machine learning (ML) is a rapidly evolving field that impacts various aspects of our lives. This FAQ section addresses common questions about machine learning, covering its definition, applications, benefits, challenges, and future implications.
What is Machine Learning?
Machine learning is a branch of artificial intelligence (AI) where algorithms learn from data and improve their performance over time without explicit programming. It allows computers to find hidden insights and patterns in data, making predictions and decisions based on that information.
What are the Types of Machine Learning?
Machine learning can be categorized into several types:
- Supervised Learning: Algorithms learn from labeled data, making predictions or classifications based on past examples.
- Unsupervised Learning: Algorithms uncover patterns in data without predefined labels, identifying hidden structures or groupings.
- Reinforcement Learning: Agents learn to make decisions by interacting with an environment and receiving rewards or penalties based on their actions.
- Semi-supervised Learning: Combines labeled and unlabeled data to improve learning accuracy.
- Deep Learning: A subset of machine learning where algorithms mimic the workings of the human brain through artificial neural networks, suitable for complex tasks like image and speech recognition.
Where is Machine Learning Used?
Machine learning finds applications across various industries and domains:
- E-commerce: Recommending products based on user preferences.
- Healthcare: Diagnosing diseases and predicting patient outcomes.
- Finance: Detecting fraud and optimizing investments.
- Transportation: Autonomous vehicles for safer driving.
- Entertainment: Personalizing content recommendations on streaming platforms.
- Manufacturing: Predictive maintenance to reduce downtime.
What are the Benefits of Machine Learning?
Machine learning offers numerous advantages:
- Improved Decision Making: Algorithms analyze data to provide insights and predictions, aiding in better decision-making processes.
- Automation: Tasks can be automated, enhancing efficiency and reducing human error.
- Personalization: Services and products can be tailored to individual preferences, enhancing user satisfaction.
- Cost Savings: Predictive maintenance and fraud detection help in saving costs by preventing failures and losses.
- Innovation: ML drives innovation in various fields, pushing the boundaries of what technology can achieve.
What are the Challenges of Machine Learning?
Machine learning also poses several challenges:
- Data Quality: ML models require large amounts of high-quality data for accurate predictions.
- Bias and Fairness: Algorithms can inherit biases present in data, leading to unfair or discriminatory outcomes.
- Interpretability: Complex models like deep learning can be difficult to interpret, making it hard to understand how decisions are made.
- Privacy Concerns: ML relies on data, raising concerns about data privacy, security, and the ethical use of personal information.
- Implementation Costs: Developing and deploying ML solutions can be expensive and require skilled professionals.
- Job Displacement: Automation driven by ML may lead to job losses in certain industries, requiring retraining and adaptation in the workforce.
How is Machine Learning Regulated?
Regulation of machine learning varies by jurisdiction and application:
- Data Protection Laws: Regulations like GDPR in Europe and CCPA in California govern how personal data is collected, stored, and used.
- Ethical Guidelines: Organizations develop ethical frameworks to ensure responsible use of AI and ML technologies.
- Industry Standards: Some industries have specific guidelines for the use of ML, ensuring safety and fairness.
What is the Future of Machine Learning?
The future of machine learning holds promising advancements:
- Advancements in AI: Continued progress in deep learning and neural networks for more complex tasks.
- Integration with IoT: ML combined with Internet of Things (IoT) devices for real-time data analysis and decision-making.
- Ethical AI: Emphasis on developing ethical AI systems that prioritize fairness, transparency, and accountability.
- Human-Machine Collaboration: Enhancing human capabilities through AI while addressing societal challenges.
- Industry-specific Applications: Tailoring ML solutions to address specific challenges in healthcare, finance, manufacturing, and more.
Conclusion
Machine learning is reshaping industries and everyday life, offering immense potential while posing significant challenges. Understanding these aspects is crucial for harnessing its benefits responsibly and navigating its complexities effectively in the evolving technological landscape.
This FAQ section covers fundamental questions about machine learning, providing insights into its definition, types, applications, benefits, challenges, regulatory aspects, and future directions. Each topic can be expanded with real-world examples, case studies, and expert opinions to provide readers with a deeper understanding of the transformative impact of machine learning.
Disclaimer and Caution: Understanding the Limitations and Risks of Machine Learning
Machine learning (ML) is a powerful tool with transformative potential across industries. However, it’s essential to acknowledge its limitations, risks, and ethical considerations. This section provides a detailed disclaimer and cautionary note to help stakeholders navigate the complexities and challenges associated with ML.
Limitations of Machine Learning
Machine learning algorithms are not without constraints:
- Data Dependency: ML models require large amounts of high-quality data for training. Insufficient or biased data can lead to inaccurate predictions or reinforce existing biases.
- Overfitting: Algorithms may perform well on training data but fail to generalize to new, unseen data. This phenomenon, known as overfitting, can result in unreliable outcomes.
- Interpretability: Complex ML models like deep neural networks are often considered “black boxes,” making it challenging to understand how decisions are made. Lack of interpretability can hinder trust and accountability.
- Performance Trade-offs: Optimizing ML models involves balancing accuracy, speed, and resource consumption. Improving one aspect may compromise others, requiring careful consideration in practical applications.
- Algorithmic Bias: ML algorithms can perpetuate biases present in training data, leading to discriminatory outcomes in sensitive areas such as hiring, lending, and law enforcement.
Ethical Considerations and Risks
Deploying machine learning involves ethical considerations:
- Privacy Concerns: ML relies on vast amounts of data, raising concerns about data privacy, security breaches, and the unauthorized use of personal information.
- Fairness and Transparency: Algorithms should be designed to ensure fairness and transparency, avoiding discriminatory outcomes and enabling stakeholders to understand decision-making processes.
- Accountability: As ML systems become more autonomous, accountability for decisions and actions becomes crucial. Clear governance frameworks and regulatory guidelines are essential to mitigate risks.
- Impact on Jobs: Automation driven by ML may disrupt industries and lead to job displacement. Addressing workforce implications through education and retraining programs is necessary.
- Bias Mitigation: Proactively identifying and mitigating biases in training data and algorithms is critical to ensure equitable outcomes and maintain public trust.
Practical Challenges and Considerations
Implementing machine learning involves navigating practical challenges:
- Skill Gap: Developing and deploying ML solutions requires expertise in data science, programming, and domain knowledge. Addressing the skill gap through training and recruitment is essential.
- Cost and Resource Intensiveness: ML projects can be expensive and resource-intensive, requiring investments in infrastructure, data management, and ongoing maintenance.
- Regulatory Compliance: Adhering to data protection laws, industry regulations, and ethical guidelines is crucial to avoid legal and reputational risks.
- Integration with Existing Systems: Integrating ML solutions with legacy systems and workflows requires careful planning and testing to ensure compatibility and minimize disruption.
- Performance Monitoring and Maintenance: Continuous monitoring of ML models’ performance and updating them as new data becomes available are essential to maintain accuracy and relevance over time.
Mitigating Risks and Ensuring Responsible Use
To harness the benefits of machine learning while mitigating risks, stakeholders should:
- Invest in Data Quality: Ensure data used for training and evaluation is comprehensive, unbiased, and up-to-date.
- Promote Transparency: Foster transparency in algorithmic decision-making processes to build trust and accountability.
- Implement Ethical Guidelines: Adhere to ethical principles and guidelines to ensure fair and responsible use of ML technologies.
- Monitor Performance: Regularly monitor and evaluate ML models’ performance to detect biases, errors, and performance degradation.
- Educate Stakeholders: Educate users, employees, and decision-makers about the capabilities, limitations, and ethical considerations of machine learning.
- Collaborate with Regulators: Work with regulators and policymakers to establish clear guidelines and regulations for the ethical use of AI and machine learning.
Conclusion
Machine learning offers tremendous potential to transform industries and improve human lives. However, understanding its limitations, risks, and ethical considerations is essential for responsible deployment and sustainable innovation. By addressing these challenges proactively, stakeholders can maximize the benefits of machine learning while minimizing its potential drawbacks, ensuring a balanced and ethical approach to its adoption.
This disclaimer and cautionary section aims to provide a comprehensive overview of the limitations, risks, and ethical considerations associated with machine learning. It emphasizes the importance of responsible use, transparency, and ongoing evaluation to mitigate potential challenges and ensure positive outcomes from ML applications.
1 thought on “Practical Examples of Machine Learning”