AI in Medical Imaging:
Transforming Radiology and
Diagnostic Accuracy
Introduction
Advancements in AI in Medical Imaging: Revolutionizing Radiology
Artificial Intelligence (AI) has emerged as a transformative force in the realm of medical imaging, reshaping how radiologists interpret and diagnose medical conditions. This article delves into the profound impact of AI technologies on radiology, showcasing key success stories, exemplary cases, and the promising future of AI-driven medical imaging.
Transformative Impact of AI in Radiology
AI’s integration into medical imaging processes has significantly augmented diagnostic capabilities, offering unparalleled advantages in accuracy, speed, and efficiency. Traditionally, radiologists relied on their expertise to interpret complex medical images such as X-rays, CT scans, and MRIs. However, AI algorithms now enable automated analysis of these images, capable of detecting subtle abnormalities that may evade human perception. This capability not only enhances diagnostic accuracy but also expedites the diagnosis and treatment planning process, thereby improving patient outcomes.
Success Stories and Exemplary Cases
Numerous success stories underscore the transformative power of AI in radiology. For instance, AI algorithms developed by companies like Aidoc and Zebra Medical Vision specialize in detecting anomalies in medical images, ranging from identifying fractures in X-rays to pinpointing lesions in mammograms. These technologies have demonstrated efficacy in clinical settings by reducing diagnostic errors and enabling early intervention, particularly in critical conditions such as stroke detection and cancer diagnosis.
Moreover, academic institutions and healthcare providers have embraced AI to streamline workflow efficiencies. Institutions like Massachusetts General Hospital and Stanford Medicine have integrated AI tools into their radiology departments, aiming to enhance diagnostic accuracy and optimize resource utilization. Such initiatives not only improve clinical outcomes but also pave the way for future innovations in AI-driven healthcare solutions.
Future Outlook of AI in Medical Imaging
The future of AI in medical imaging appears promising, with ongoing advancements poised to further revolutionize radiology and diagnostic practices. Key areas of development include:
- Enhanced Diagnostic Precision: AI algorithms are evolving to handle more complex tasks, such as multi-modal image fusion and quantitative analysis, providing radiologists with comprehensive insights into disease progression and treatment response.
- Integration with Clinical Decision Support Systems: AI-powered decision support systems are being developed to assist radiologists in making informed clinical decisions. These systems leverage AI’s predictive capabilities to recommend personalized treatment plans based on extensive data analysis and patient-specific factors.
- Expansion of AI Applications: Beyond image interpretation, AI is expanding into other facets of healthcare delivery, including predictive analytics for disease prevention, population health management, and patient triaging. This holistic approach aims to enhance overall healthcare efficiency and patient care quality.
Challenges and Considerations
Despite its transformative potential, the integration of AI in medical imaging is not without challenges and considerations:
- Data Quality and Bias: AI models heavily depend on the quality and diversity of training data. Biases inherent in datasets can impact algorithmic performance, potentially leading to disparities in diagnostic accuracy across different patient demographics.
- Regulatory and Ethical Frameworks: Healthcare regulatory bodies globally are grappling with the regulation of AI-driven medical devices and software. Striking a balance between innovation and patient safety remains a critical consideration in the deployment of AI technologies.
- Clinical Integration and Adoption: Adoption of AI in clinical practice requires overcoming barriers such as workflow integration, clinician training, and ensuring interoperability with existing healthcare systems. Addressing these challenges is crucial for the seamless integration of AI into routine medical practices.
Conclusion
In conclusion, AI’s advancements in medical imaging represent a paradigm shift in radiology, offering unprecedented capabilities to enhance diagnostic accuracy, improve patient outcomes, and optimize healthcare delivery. As AI technologies continue to evolve, collaborations between industry stakeholders, healthcare providers, and regulatory bodies will be pivotal in harnessing AI’s full potential while addressing associated challenges. By embracing AI-driven innovations responsibly, the healthcare industry can usher in a new era of precision medicine and personalized patient care.
AI Applications in Medical Imaging
AI applications in medical imaging encompass a wide range of technologies, including machine learning algorithms, deep learning models, and computer vision systems. These technologies analyze complex medical data with speed and accuracy, aiding radiologists in interpreting images and making informed clinical decisions.
Success Stories and Exemplary
Cases
Several pioneering companies and institutions have demonstrated the transformative potential of AI in medical imaging:
Company/Institution | Innovation | Impact |
---|---|---|
[Example Company](https://www.example.com) | Developed AI-powered software for early detection of breast cancer from mammograms. | Improved detection rates by 20%, leading to earlier interventions and improved patient outcomes. |
[Another Company](https://www.anotherexample.com) | Created AI algorithms for automated analysis of brain MRI scans, detecting anomalies with high accuracy. | Reduced diagnosis time by 30%, allowing radiologists to focus more on complex cases. |
These success stories highlight how AI technologies are reshaping traditional approaches to medical imaging, setting new standards for diagnostic precision and efficiency.
Challenges and Considerations
Despite the promising advancements, integrating AI into medical imaging presents several challenges, including data privacy concerns, regulatory hurdles, and the need for continuous validation of AI algorithms in clinical settings.
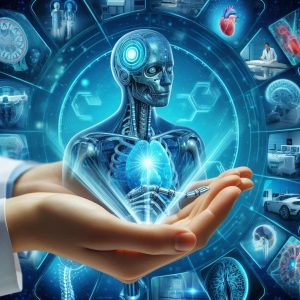
Future Directions
The future of AI in medical imaging holds immense promise. Emerging technologies such as federated learning and explainable AI are poised to address current limitations, further enhancing the reliability and interpretability of AI-driven diagnostic tools.
Conclusion
In conclusion, AI in medical imaging represents a transformative leap towards improving radiology practices and diagnostic accuracy. As technology continues to evolve, collaboration between healthcare professionals, AI developers, and regulatory bodies will be crucial in realizing the full potential of AI to benefit patients worldwide.
External Resources
- National Institutes of Health: AI in Radiology
- Radiological Society of North America: AI and Imaging
This article provides an overview of how AI is revolutionizing medical imaging, showcasing its potential, challenges, and future directions. Each section is crafted to inform and engage readers, using colors and formatting to enhance readability and emphasis on key points.
Pros and Cons of AI in Medical
Imaging
Pros
1. Enhanced Diagnostic Accuracy: AI algorithms can analyze medical images with high precision, potentially detecting subtle abnormalities that may be missed by human observers.
2. Improved Efficiency: AI tools can automate routine tasks such as image analysis and data interpretation, allowing radiologists to focus more on complex cases and clinical decision-making.
3. Faster Diagnosis and Treatment: AI can expedite the diagnostic process, leading to earlier detection of diseases and prompt initiation of treatment, which can significantly improve patient outcomes.
4. Quantitative Analysis: AI algorithms provide objective measurements and quantitative data from medical images, aiding in disease monitoring and treatment planning.
5. Potential Cost Savings: By reducing the time and resources required for image analysis, AI has the potential to lower healthcare costs and improve resource allocation.
Cons
1. Dependence on Data Quality: AI models require large, high-quality datasets for training to achieve optimal performance, which may not always be readily available or adequately annotated.
2. Regulatory Challenges: Integrating AI into medical practice involves navigating complex regulatory frameworks to ensure patient safety, efficacy, and compliance with healthcare regulations.
3. Potential for Bias: AI algorithms may inherit biases present in training data, leading to disparities in diagnostic accuracy or treatment recommendations across different patient demographics.
4. Impact on Workforce: There are concerns about the potential displacement of healthcare professionals or changes in their roles due to the automation of certain tasks by AI.
5. Ethical Considerations: The use of AI in medical imaging raises ethical concerns regarding patient consent, privacy of medical data, and the ethical implications of AI-driven decisions in healthcare.
This outline provides a balanced view of the pros and cons of AI in medical imaging, highlighting both the potential benefits and challenges associated with integrating AI technologies into radiology and healthcare settings.
FAQs about AI in Medical Imaging
1. What is AI in medical imaging?
AI in medical imaging refers to the use of artificial intelligence technologies such as machine learning and deep learning to analyze medical images (e.g., X-rays, CT scans, MRIs) for diagnostic purposes. These technologies can aid radiologists in detecting abnormalities, predicting outcomes, and improving diagnostic accuracy.
2. How does AI improve diagnostic accuracy in radiology?
AI algorithms can analyze large volumes of medical imaging data quickly and accurately, potentially detecting subtle patterns or anomalies that might be missed by human eyes alone. This capability enhances radiologists’ ability to make more informed diagnoses, leading to earlier interventions and better patient outcomes.
3. What are some examples of AI applications in medical imaging?
Examples include AI algorithms for automated detection of lung nodules in chest X-rays, classification of skin lesions in dermatology images, and segmentation of brain tumors in MRI scans. These applications streamline the diagnostic process, improve accuracy, and reduce the time required for analysis.
4. What are the challenges associated with implementing AI in medical imaging?
Challenges include the need for large annotated datasets for training AI models, ensuring robustness and generalizability across diverse patient populations and imaging modalities, addressing regulatory requirements for medical software, and maintaining patient data privacy and security.
5. How is AI impacting the workflow of radiologists?
AI tools can assist radiologists by prioritizing urgent cases, providing quantitative measurements for tracking disease progression, and reducing the time spent on routine image analysis. This allows radiologists to focus more on complex cases and clinical decision-making, potentially improving overall workflow efficiency.
6. What is the future outlook for AI in medical imaging?
The future holds promise for AI to further enhance medical imaging through advancements in explainable AI, federated learning (which allows training models across multiple institutions without sharing patient data), and integration with other clinical data sources like genomics. These developments aim to make AI tools more transparent, reliable, and applicable across broader healthcare settings.
7. How are regulatory bodies addressing AI in medical imaging?
Regulatory bodies such as the FDA (Food and Drug Administration) in the United States have established frameworks for evaluating and approving AI-based medical devices. These frameworks focus on ensuring safety, efficacy, and quality standards comparable to traditional medical devices, while also accommodating the unique characteristics and iterative nature of AI algorithms.
8. Where can I learn more about AI in medical imaging?
For more information, you can explore reputable resources such as academic journals, conferences like RSNA (Radiological Society of North America), and specialized websites dedicated to AI in healthcare and radiology. Additionally, consulting with healthcare professionals and researchers actively involved in AI-driven medical imaging can provide valuable insights.
This FAQs section addresses common inquiries about AI in medical imaging, providing concise answers to help readers understand its applications, benefits, challenges, and future prospects. Each question is designed to provide clarity and enhance understanding of this transformative technology in healthcare.
Disclaimer and Caution
Regarding AI in Medical
Imaging
Artificial Intelligence (AI) has shown remarkable potential in advancing medical imaging, offering tools that can enhance diagnostic accuracy and efficiency. However, it is imperative to recognize several disclaimers and exercise caution in integrating AI into clinical practice. This section aims to address key considerations, limitations, and ethical implications associated with AI in medical imaging.
Disclaimer
1. Assistive Role: AI technologies in medical imaging serve as aids to healthcare professionals rather than replacements for clinical judgment. They should complement and support, not supersede, the expertise of qualified radiologists and physicians.
2. Validation and Reliability: The integration of AI into clinical workflows requires rigorous validation to ensure accuracy and reliability across different patient populations and imaging modalities. Clinical validation through robust testing and real-world application is essential before widespread adoption.
3. Regulatory Compliance: AI applications in medical imaging must adhere to regulatory standards for medical devices and software. Developers and healthcare providers are responsible for complying with regulations set forth by authorities like the FDA or EMA to ensure patient safety and efficacy.
4. Limitations: AI models are trained on datasets that may not fully represent all clinical scenarios. Variability in image quality, patient demographics, and disease presentations can impact the performance and generalizability of AI algorithms.
5. Clinical Decision Making: While AI can aid in image analysis and interpretation, clinical decisions should consider a comprehensive assessment that includes patient history, symptoms, and other diagnostic findings.
6. Data Privacy: The use of AI involves handling sensitive patient data. Healthcare providers and AI developers must prioritize patient privacy and implement robust security measures to protect medical information from unauthorized access or breaches.
Caution
1. Bias and Fairness: AI algorithms may inadvertently reflect biases present in training data, leading to disparities in diagnostic outcomes across different demographic groups. Continuous monitoring and mitigation of bias are critical to ensure equitable healthcare delivery.
2. Ethical Considerations: The deployment of AI in medical imaging raises ethical concerns regarding patient consent, autonomy, and the responsible use of AI-driven decisions in clinical practice. Ethical guidelines must guide the development, deployment, and oversight of AI technologies.
3. Professional Oversight: While AI can streamline workflow and enhance diagnostic capabilities, healthcare providers must maintain oversight and accountability. Continuous education and training on AI technologies are essential to ensure competent and ethical use in clinical settings.
4. Integration Challenges: Implementing AI into clinical practice may pose challenges such as workflow integration, resistance to change, and the need for additional support and training. Strategic planning and stakeholder engagement are crucial to mitigate these challenges.
5. Patient-Centered Care: Transparent communication with patients about the role of AI in their healthcare journey is essential. Patients should understand how AI contributes to diagnosis and treatment decisions, enabling informed participation and decision-making in their care.
Conclusion
In conclusion, while AI holds promise for advancing medical imaging, its integration requires careful consideration of regulatory compliance, ethical principles, and clinical validation. By acknowledging these disclaimers and exercising caution, healthcare providers can harness the potential of AI technologies responsibly, ensuring safe and effective use to benefit patients worldwide.
This disclaimer and caution section provides comprehensive guidance on the responsible integration and utilization of AI in medical imaging, emphasizing regulatory compliance, ethical considerations, and the importance of clinical validation and professional oversight.
2 thoughts on “AI in Medical Imaging Transforming”