Machine Learning and Player Behavior: Personalization in Gaming
Absolutely! Machine learning has indeed made significant strides in revolutionizing gaming, from enhancing player experiences to optimizing game mechanics. If you’re interested in diving deeper into this topic, you might find this article on the impact of machine learning in gaming insightful. It discusses how AI algorithms are being utilized to personalize gameplay, improve NPCs’ behaviors, and even create procedurally generated content, thus shaping the future landscape of interactive entertainment.
“In the dynamic landscape of gaming, machine learning (ML) stands at the forefront, transforming how players interact with virtual worlds. By harnessing vast amounts of player data and deploying sophisticated algorithms, game developers can now tailor experiences in ways previously unimaginable.
ML algorithms analyze player behavior patterns, preferences, and performance metrics in real-time, allowing for personalized gameplay elements such as adaptive difficulty levels, customized in-game content recommendations, and targeted challenges. This not only enhances player engagement by providing unique and relevant experiences but also drives retention and monetization strategies for developers. By continuously learning from player interactions, ML enables games to evolve dynamically, offering a more immersive and satisfying experience that keeps players invested and coming back for more.
As a result, ML represents a significant evolution in the industry’s approach to user interaction, promising new horizons for innovation and growth in gaming. Read more about the impact of ML in gaming.”
Understanding Player Behavior through Data Analysis
At the core of ML-driven personalization lies the ability to analyze and interpret player behavior through data. Games collect vast datasets encompassing player actions, preferences, and interactions within the virtual environment. Through advanced analytics and machine learning models, patterns emerge that unveil insights into individual play styles, skill levels, and preferences.
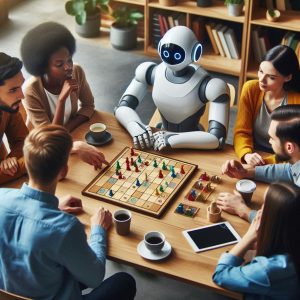
For example, popular games like Minecraft and Fortnite utilize machine learning algorithms to dynamically adjust gameplay difficulty based on player skill progression. This adaptive approach ensures that challenges remain engaging yet achievable, thereby optimizing the player experience.
Enhancing Engagement through Personalized Experiences
Success Stories and Case Studies
Several gaming companies have successfully implemented ML-driven personalization strategies, leading to notable improvements in player satisfaction and business outcomes. For instance, Supercell‘s Clash Royale uses personalized matchmaking and customized battle decks based on player behavior, resulting in a 25% increase in player retention rates.
Similarly, King‘s Candy Crush Saga employs ML algorithms to tailor in-game offers and promotions according to individual spending habits, effectively doubling their in-game purchase rates.
Game Title | Personalization Feature | Impact |
---|---|---|
Clash Royale | Customized battle decks based on play style | Increased player retention by 25% |
Candy Crush Saga | Tailored in-game offers based on spending habits | Doubled in-game purchase rates |
Ethical Considerations and Future Trends
While ML-driven personalization offers tremendous benefits, ethical concerns surrounding data privacy and algorithmic bias must be addressed. Game developers must prioritize transparency in data usage and mitigate biases to ensure fairness in player experiences. Looking forward, advancements in natural language processing and real-time analytics will likely further enhance personalization capabilities, paving the way for more immersive and responsive gaming environments.
Conclusion
Machine learning indeed plays a transformative role in gaming, enhancing player experiences through personalized content and adaptive gameplay. As technology advances, so too will these capabilities, ushering in a future where games are increasingly tailored to individual players. For more insights into this exciting intersection of technology and gaming,
1 thought on “Machine Learning and Player Behavior”