Machine Learning in 2024: Breakthroughs, Trends, and Emerging Technologies
As we navigate through 2024, the landscape of machine learning (ML) continues to evolve at an unprecedented pace. Innovations and breakthroughs are shaping industries and redefining the boundaries of what is possible. This article explores the latest trends, breakthrough technologies, and the future outlook of machine learning.
Breakthroughs in Machine Learning
2024 has witnessed several significant advancements in machine learning, driving the technology to new heights. Key breakthroughs include:
Breakthrough | Description | Impact |
---|---|---|
Generative AI Models | Advanced generative models, such as GPT-4 and beyond, are creating highly realistic text, images, and videos. | Enhanced content creation, personalized user experiences, and new creative possibilities. |
Self-Supervised Learning | New techniques in self-supervised learning reduce the need for labeled data by allowing models to learn from unlabeled data. | Improved efficiency in training ML models and reduced data labeling costs. |
AI for Drug Discovery | Machine learning algorithms are accelerating drug discovery by predicting molecular interactions and drug efficacy. | Faster development of new drugs and therapies, leading to better healthcare outcomes. |
Trends Shaping Machine Learning
Several key trends are driving the future of machine learning in 2024:
- Edge AI: The rise of edge computing is pushing machine learning capabilities to the edge of networks, enabling real-time data processing and reducing latency.
- Explainable AI (XAI): There is a growing emphasis on developing AI systems that offer transparency and explainability, enhancing trust and accountability in machine learning applications.
- AI Ethics and Governance: The focus on ethical considerations and governance frameworks for AI is increasing, addressing concerns related to bias, privacy, and fairness.
- Integration with IoT: Machine learning is increasingly being integrated with the Internet of Things (IoT) to enable smart devices and systems with predictive and adaptive capabilities.
- Automated Machine Learning (AutoML): Tools and platforms that automate various aspects of the machine learning lifecycle are making it easier for non-experts to build and deploy models.
Emerging Technologies in Machine Learning
The landscape of emerging technologies in machine learning is vibrant and diverse. Some notable technologies to watch in 2024 include:
Technology | Description | Applications |
---|---|---|
Quantum Machine Learning | Combines quantum computing with machine learning techniques to solve complex problems more efficiently. | Optimization, cryptography, and advanced data analysis. |
Neurosymbolic AI | Integrates neural networks with symbolic reasoning to improve AI’s ability to understand and reason about the world. | Complex problem solving, natural language understanding, and reasoning tasks. |
Federated Learning | Allows models to be trained across decentralized devices while keeping data local, enhancing privacy and security. | Healthcare, finance, and personalized services. |
Success Stories and Exemplary Cases
Numerous organizations and projects are exemplifying the transformative power of machine learning. Here are a few notable success stories:
1. NVIDIA has revolutionized AI with its advanced GPUs and software frameworks, powering cutting-edge research and applications across various domains.
2. DeepMind continues to push the boundaries of AI with breakthroughs in protein folding and game-playing AI, showcasing the potential of machine learning in solving real-world problems.
3. C3.ai offers AI-driven solutions for enterprise-scale applications, demonstrating the impact of machine learning on business operations and decision-making.
4. OpenAI has made headlines with its powerful language models and innovations in reinforcement learning, setting new standards in natural language processing and AI research.
Challenges and Future Outlook
Despite the impressive advancements, machine learning in 2024 faces several challenges:
- Data Privacy: Ensuring the protection of sensitive data used in training machine learning models remains a critical concern.
- Bias and Fairness: Addressing biases in algorithms and ensuring fairness in machine learning outcomes is crucial for building trustworthy systems.
- Scalability: Scaling machine learning models and infrastructure to handle large volumes of data efficiently poses technical and logistical challenges.
- Integration with Existing Systems: Integrating new machine learning technologies with legacy systems can be complex and requires careful planning and execution.
Looking ahead, the future of machine learning holds immense promise. Continued research and development are expected to drive further breakthroughs, enabling more sophisticated and impactful applications across diverse fields. For more information on the latest trends and technologies in machine learning, you can explore resources from reputable sources:
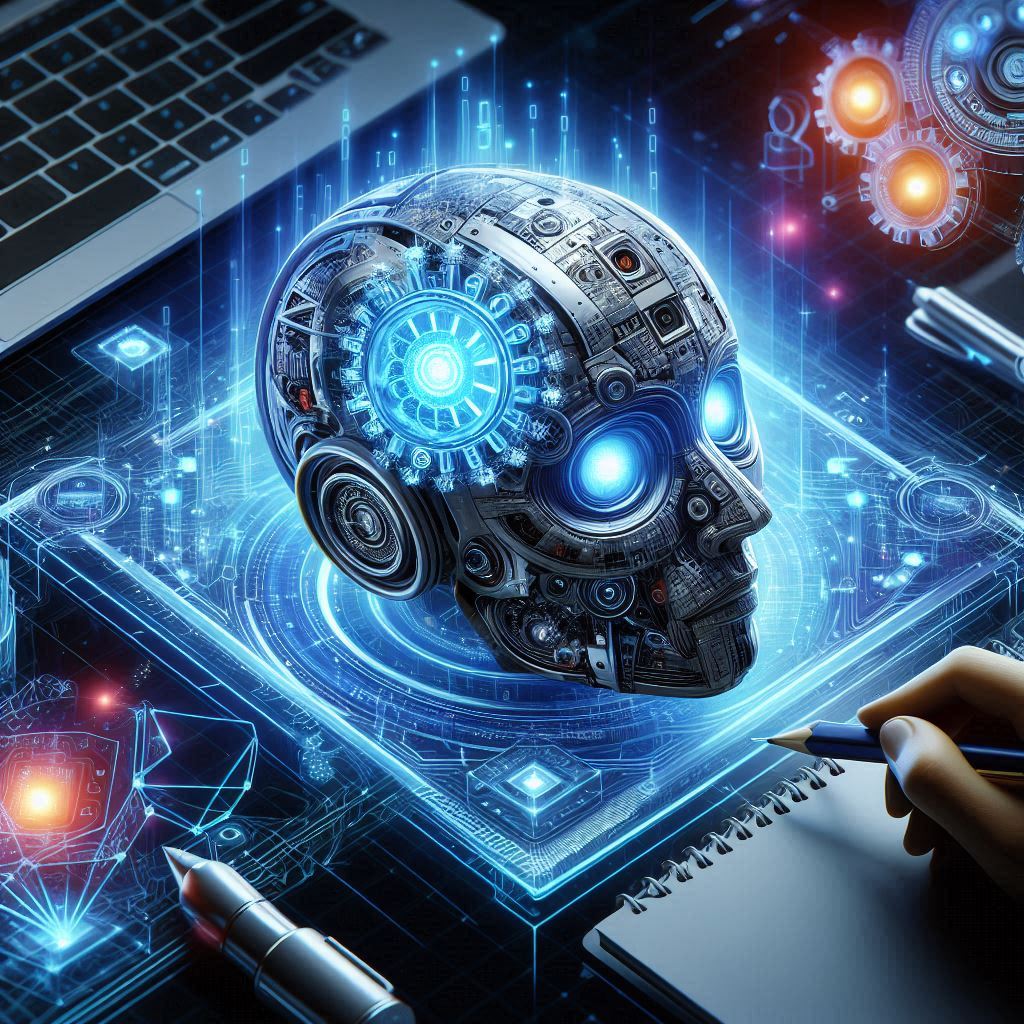
Pros and Cons of Machine Learning in 2024
Machine learning (ML) has rapidly evolved over the years, with its applications becoming increasingly integral to various industries. As of 2024, the technology has reached new heights, offering numerous benefits while also presenting certain challenges. This article delves into the pros and cons of machine learning, providing a balanced perspective on its impact and implications.
Pros of Machine Learning in 2024
1. Enhanced Efficiency and Productivity
Machine learning algorithms can process vast amounts of data much faster and more accurately than humans. This capability translates into significant improvements in efficiency and productivity across various sectors:
- Automation: ML automates repetitive tasks, reducing the need for manual intervention and freeing up human resources for more complex activities.
- Data Analysis: Advanced ML models can analyze large datasets to extract valuable insights, facilitating informed decision-making.
2. Improved Personalization
Machine learning enhances user experiences through personalization. By analyzing user behavior and preferences, ML models can tailor content and services to individual needs:
- Marketing: Personalized advertising and recommendations increase user engagement and conversion rates.
- Healthcare: Personalized treatment plans and predictive analytics improve patient outcomes and streamline healthcare delivery.
3. Innovation and New Capabilities
ML drives innovation by enabling new capabilities and applications that were previously unimaginable:
- Generative Models: ML models like GPT-4 and DALL-E are creating highly realistic text, images, and videos, expanding creative possibilities.
- Autonomous Systems: Advances in ML have paved the way for autonomous vehicles and drones, revolutionizing transportation and logistics.
4. Enhanced Decision-Making
ML algorithms can support decision-making processes by providing accurate predictions and recommendations based on data analysis:
- Financial Services: ML models help in fraud detection, risk management, and investment strategies.
- Manufacturing: Predictive maintenance and quality control improve operational efficiency and reduce downtime.
Cons of Machine Learning in 2024
1. Data Privacy and Security Concerns
As ML systems rely heavily on data, concerns about privacy and security are prominent:
- Data Breaches: The accumulation of vast amounts of personal data increases the risk of data breaches and unauthorized access.
- Privacy Invasion: The use of personal data for training ML models can lead to concerns about privacy invasion and misuse.
2. Bias and Fairness Issues
ML algorithms can inadvertently perpetuate biases present in training data, leading to unfair outcomes:
- Algorithmic Bias: Bias in training data can result in discriminatory practices and reinforce existing inequalities.
- Transparency: The “black box” nature of some ML models makes it challenging to understand and rectify biased decisions.
3. High Costs and Resource Requirements
Developing and deploying ML systems can be costly and resource-intensive:
- Infrastructure Costs: Training large ML models requires significant computational resources and infrastructure investment.
- Expertise: Specialized skills and expertise are needed to develop, implement, and maintain ML systems, which can be a barrier for smaller organizations.
4. Ethical and Social Implications
The use of ML raises various ethical and social concerns:
- Job Displacement: Automation and ML-driven processes can lead to job displacement and affect employment opportunities in certain sectors.
- Decision-Making Accountability: Determining accountability for decisions made by ML systems can be complex, especially in critical applications like healthcare and criminal justice.
Balancing the Pros and Cons
While machine learning offers numerous advantages, it is crucial to address the associated challenges to maximize its benefits. Here are some strategies to balance the pros and cons:
- Implement Robust Data Privacy Measures: Organizations should adopt stringent data privacy practices and ensure compliance with regulations to protect user data.
- Focus on Fairness and Transparency: Developing fair and transparent ML models involves regular auditing, bias mitigation strategies, and stakeholder engagement.
- Invest in Training and Education: Providing training and resources to upskill workers can help mitigate the impact of job displacement and enhance the overall workforce capability.
- Promote Ethical AI Practices: Establishing ethical guidelines and frameworks for ML development and deployment can address ethical concerns and ensure responsible use of technology.
For further reading and insights on machine learning and its impact, you can explore resources from reputable sources:
This article highlights the key advantages and challenges associated with machine learning, offering a comprehensive view of its impact in 2024. It also provides strategies for addressing the challenges to maximize the benefits of ML technologies.
Frequently Asked Questions (FAQs) on Machine Learning in 2024
1. What is Machine Learning?
Machine Learning (ML) is a subset of artificial intelligence (AI) that enables systems to learn from data and improve their performance over time without being explicitly programmed. ML algorithms identify patterns in data, make predictions, and make decisions based on new data inputs.
For more details, visit IBM – What is Machine Learning?
2. What are the key breakthroughs in Machine Learning for 2024?
In 2024, several key breakthroughs have significantly advanced the field of machine learning:
- Generative AI Models: Technologies such as GPT-4 and DALL-E are pushing the boundaries of content creation by generating realistic text, images, and videos.
- Self-Supervised Learning: This approach reduces the dependency on labeled data by allowing models to learn from vast amounts of unlabeled data.
- AI for Drug Discovery: ML models are accelerating the drug discovery process by predicting molecular interactions and drug efficacy.
Learn more about these breakthroughs at TechCrunch – Machine Learning
3. How does Machine Learning impact various industries?
Machine learning has a profound impact on multiple industries, enhancing efficiency and innovation:
- Healthcare: ML improves diagnostics, personalizes treatment, and optimizes hospital operations.
- Finance: It aids in fraud detection, risk management, and investment strategies.
- Retail: ML enables personalized recommendations, inventory management, and customer insights.
- Manufacturing: Predictive maintenance and quality control are optimized through ML algorithms.
For industry-specific insights, check Forbes – Machine Learning Trends
4. What are the main challenges associated with Machine Learning?
Despite its advantages, machine learning faces several challenges:
- Data Privacy and Security: The accumulation of personal data raises concerns about privacy breaches and unauthorized access.
- Bias and Fairness: ML models can perpetuate biases present in training data, leading to unfair outcomes.
- High Costs: Developing and maintaining ML systems can be resource-intensive, requiring significant computational power and expertise.
- Ethical Issues: ML applications can lead to ethical dilemmas, such as job displacement and accountability for automated decisions.
Explore detailed discussions on these challenges at BBC – Machine Learning Updates
5. What is Explainable AI (XAI) and why is it important?
Explainable AI (XAI) refers to methods and techniques that make the outputs of machine learning models understandable to humans. XAI aims to address the “black box” problem where complex models operate in ways that are not transparent:
- Transparency: XAI provides insights into how decisions are made by ML models.
- Trust: By understanding model decisions, stakeholders are more likely to trust and adopt ML systems.
- Accountability: XAI facilitates accountability by allowing organizations to identify and correct biases or errors in model predictions.
Read more about XAI at CNN – Machine Learning Trends
6. How is Machine Learning integrated with the Internet of Things (IoT)?
Machine learning enhances IoT by enabling smart devices to make decisions based on data from their environment. This integration allows for:
- Real-Time Analytics: ML algorithms process data from IoT sensors in real-time, enabling immediate responses and actions.
- Predictive Maintenance: IoT devices equipped with ML can predict equipment failures and schedule maintenance before problems occur.
- Enhanced Automation: ML-powered IoT systems can automate complex processes and adapt to changing conditions.
Discover more about IoT and ML integration at Wired – Machine Learning Insights
7. What are Automated Machine Learning (AutoML) tools and how do they benefit non-experts?
Automated Machine Learning (AutoML) tools simplify the ML development process by automating tasks such as model selection, hyperparameter tuning, and feature engineering:
- User-Friendly Interfaces: AutoML platforms provide intuitive interfaces that allow users to build and deploy ML models without extensive expertise.
- Efficiency: AutoML reduces the time and effort required to develop machine learning models, accelerating project timelines.
- Accessibility: These tools make advanced ML techniques accessible to a broader audience, including those with limited technical backgrounds.
For more information on AutoML, visit MIT Technology Review – Machine Learning 2024
8. What are the future trends in Machine Learning?
The future of machine learning is marked by several emerging trends:
- Quantum Machine Learning: Integrating quantum computing with ML to solve complex problems more efficiently.
- Neurosymbolic AI: Combining neural networks with symbolic reasoning for improved understanding and reasoning.
- Federated Learning: Training models across decentralized devices while keeping data local to enhance privacy and security.
Stay updated with future trends at Science Daily – Machine Learning News
9. How can organizations address the ethical issues related to Machine Learning?
Addressing ethical issues in machine learning involves several strategies:
- Develop Ethical Guidelines: Establish clear ethical guidelines for ML development and deployment to ensure responsible use of technology.
- Implement Bias Mitigation Strategies: Regularly audit ML models for biases and employ techniques to mitigate them.
- Promote Transparency and Accountability: Ensure transparency in ML processes and maintain accountability for automated decisions.
Learn more about ethical practices in AI at Forbes – Machine Learning Ethics
Disclaimer and Caution
Machine learning (ML) is a rapidly evolving field with significant advancements and applications across various domains. However, it is crucial to understand the limitations, potential risks, and ethical considerations associated with these technologies. This disclaimer and caution section aims to provide a detailed overview to ensure informed and responsible use of machine learning systems.
1. Accuracy and Reliability
While machine learning models can offer valuable insights and predictions, their accuracy and reliability can vary significantly based on several factors:
- Data Quality: The performance of ML models heavily relies on the quality and completeness of the data used for training. Inaccurate, incomplete, or biased data can lead to unreliable or misleading results.
- Model Limitations: ML models are designed based on specific algorithms and training data. They may not perform well outside the conditions they were trained for or in novel situations.
- Validation: Continuous validation and testing are essential to ensure that ML models maintain their accuracy and relevance over time. Regular updates and retraining are necessary to adapt to changing conditions.
For more information on data quality and model validation, visit O’Reilly – Machine Learning Guide
2. Data Privacy and Security
Machine learning systems often process vast amounts of personal and sensitive data. Ensuring data privacy and security is paramount:
- Data Protection: Organizations must implement robust measures to protect personal data from unauthorized access and breaches. Compliance with data protection regulations, such as GDPR or CCPA, is essential.
- Data Anonymization: Anonymizing data can reduce privacy risks by removing personally identifiable information, but it may also affect the model’s performance.
- Ethical Use: Ethical considerations should guide the collection and use of data, ensuring that data is obtained with consent and used responsibly.
Learn more about data protection and privacy at EU GDPR Information Portal
3. Bias and Fairness
Machine learning models can inadvertently perpetuate or amplify biases present in training data, leading to unfair or discriminatory outcomes:
- Algorithmic Bias: Biases in training data can result in biased predictions or decisions. It is crucial to identify and address these biases to prevent discriminatory outcomes.
- Fairness: Ensuring fairness in ML models involves assessing and mitigating biases and striving for equitable treatment across different demographic groups.
- Transparency: Transparency in model development and decision-making processes helps stakeholders understand and address potential biases.
For resources on addressing bias in machine learning, visit Microsoft Research – Understanding Bias in AI
4. Ethical and Social Implications
The deployment of machine learning technologies can have significant ethical and social implications:
- Job Displacement: Automation and ML-driven processes may lead to job displacement, affecting employment opportunities in certain sectors. Organizations should consider reskilling and upskilling initiatives to support affected workers.
- Accountability: Determining accountability for decisions made by ML systems can be challenging, especially in critical areas like healthcare and criminal justice.
- Ethical Use: Ethical guidelines and frameworks should govern the development and deployment of ML technologies to ensure responsible use and mitigate negative impacts.
Explore more about the ethical implications of AI at CNN – Ethics in AI
5. Dependence on Technology
Heavy reliance on machine learning systems can lead to over-dependence on technology, which may have consequences:
- Decision-Making: Over-reliance on ML models for decision-making may result in reduced human oversight and critical thinking, potentially leading to errors or unintended consequences.
- System Failures: Technical issues, system failures, or unexpected behavior in ML models can disrupt operations and impact outcomes.
- Adaptability: Organizations must ensure that their systems are adaptable and that they maintain human oversight to address situations beyond the scope of automated systems.
Read more about managing technological dependence at Forbes – Technology Dependence
6. Regulatory and Legal Considerations
Machine learning technologies are subject to various regulatory and legal considerations:
- Compliance: Organizations must comply with relevant regulations and standards governing data use, privacy, and ethical considerations. This includes adhering to regulations such as GDPR, CCPA, and industry-specific guidelines.
- Legal Risks: Failure to comply with legal requirements can result in legal actions, fines, and reputational damage.
- Regulatory Changes: The regulatory landscape for ML and AI is evolving. Organizations should stay informed about changes in regulations and adapt their practices accordingly.
For information on AI regulations and compliance, visit Wired – AI Regulations
7. Recommendations for Responsible Use
To ensure the responsible use of machine learning technologies, consider the following recommendations:
- Implement Ethical Guidelines: Develop and adhere to ethical guidelines for the use of ML technologies, addressing issues related to fairness, transparency, and accountability.
- Ensure Data Integrity: Use high-quality, representative data for training ML models and implement measures to protect data privacy and security.
- Promote Transparency: Make the workings of ML models transparent to stakeholders, including how decisions are made and how biases are addressed.
- Maintain Human Oversight: Ensure that human oversight is maintained in decision-making processes involving ML technologies to mitigate potential risks and errors.
- Stay Informed: Keep up-to-date with advancements in ML, regulatory changes, and best practices to adapt to evolving challenges and opportunities.
For best practices and guidelines on responsible AI use, visit MIT Technology Review – Responsible AI Use
In summary, while machine learning offers transformative capabilities and opportunities, it is essential to approach its use with caution and responsibility. By understanding the limitations, addressing ethical concerns, and adhering to best practices, organizations and individuals can harness the power of ML while mitigating potential risks.
This disclaimer and caution section provides a comprehensive overview of the various considerations related to machine learning in 2024, helping users understand the technology’s limitations and ethical implications while offering guidance on responsible use.