The Evolution of Machine Learning: Key Developments and Future Prospects
Introduction
Machine learning (ML) has undergone remarkable transformations since its inception. Initially a niche area within artificial intelligence (AI), it has now become a cornerstone of technological advancements. This article explores the evolution of machine learning, highlights key developments, and discusses future prospects.
Early Milestones in Machine Learning
Machine learning has its roots in the 1950s and 1960s, with early work by pioneers such as Arthur Samuel and Frank Rosenblatt. Samuel’s work on self-learning algorithms and Rosenblatt’s development of the perceptron laid the foundation for modern ML techniques.
The Rise of Neural Networks and Deep Learning
The 1980s and 1990s saw significant advancements in neural networks. The introduction of the backpropagation algorithm allowed for more efficient training of deep networks. This period also saw the emergence of deep learning, a subset of machine learning that uses algorithms known as neural networks to model complex patterns in data.
Breakthroughs in Machine Learning
Several key breakthroughs have propelled machine learning into the mainstream. Notable developments include:
- Support Vector Machines (SVMs): Introduced in the 1990s, SVMs became popular for classification tasks.
- Convolutional Neural Networks (CNNs): CNNs revolutionized image recognition with their ability to process pixel data effectively.
- Recurrent Neural Networks (RNNs): RNNs excel in sequence prediction, which is crucial for tasks like natural language processing.
Success Stories and Exemplary Cases
Numerous advancements in machine learning have been exemplified by groundbreaking companies and research projects. For instance:
- Google AI has made significant contributions to natural language understanding and computer vision.
- OpenAI has been at the forefront of developing large language models and reinforcement learning systems.
- IBM Watson has been applied to various domains including healthcare and finance, showcasing the versatility of ML applications.
Machine Learning in Different Industries
Machine learning is transforming various industries through applications such as:
Industry | Application | Impact |
---|---|---|
Healthcare | Predictive Analytics | Improved diagnostics and personalized treatment plans. |
Finance | Fraud Detection | Enhanced security and reduced fraudulent activities. |
Retail | Recommendation Systems | Increased sales through personalized shopping experiences. |
Challenges and Ethical Considerations
Despite its advancements, machine learning faces several challenges:
- Bias and Fairness: Ensuring algorithms are free from bias is critical for equitable outcomes. Addressing bias is a major focus of current research.
- Privacy: Machine learning models often require large amounts of data, raising concerns about data privacy and security.
- Explainability: Understanding how models make decisions is essential for trust and transparency. Explainable AI is an emerging field aimed at addressing this issue.
Future Prospects and Emerging Trends
The future of machine learning is poised for exciting developments. Key trends include:
- Federated Learning: This approach allows models to be trained across decentralized data sources while keeping data localized, enhancing privacy and security.
- Advancements in Deep Learning: Innovations in deep learning architectures are expected to drive more sophisticated AI applications.
- Ethical AI: Future developments will likely focus on creating more ethical and unbiased AI systems.
Conclusion
The evolution of machine learning is a testament to its transformative potential across various domains. As technology advances, machine learning will continue to shape the future, addressing challenges and embracing new opportunities. Staying informed about these developments will be crucial for harnessing the power of machine learning in the coming years.
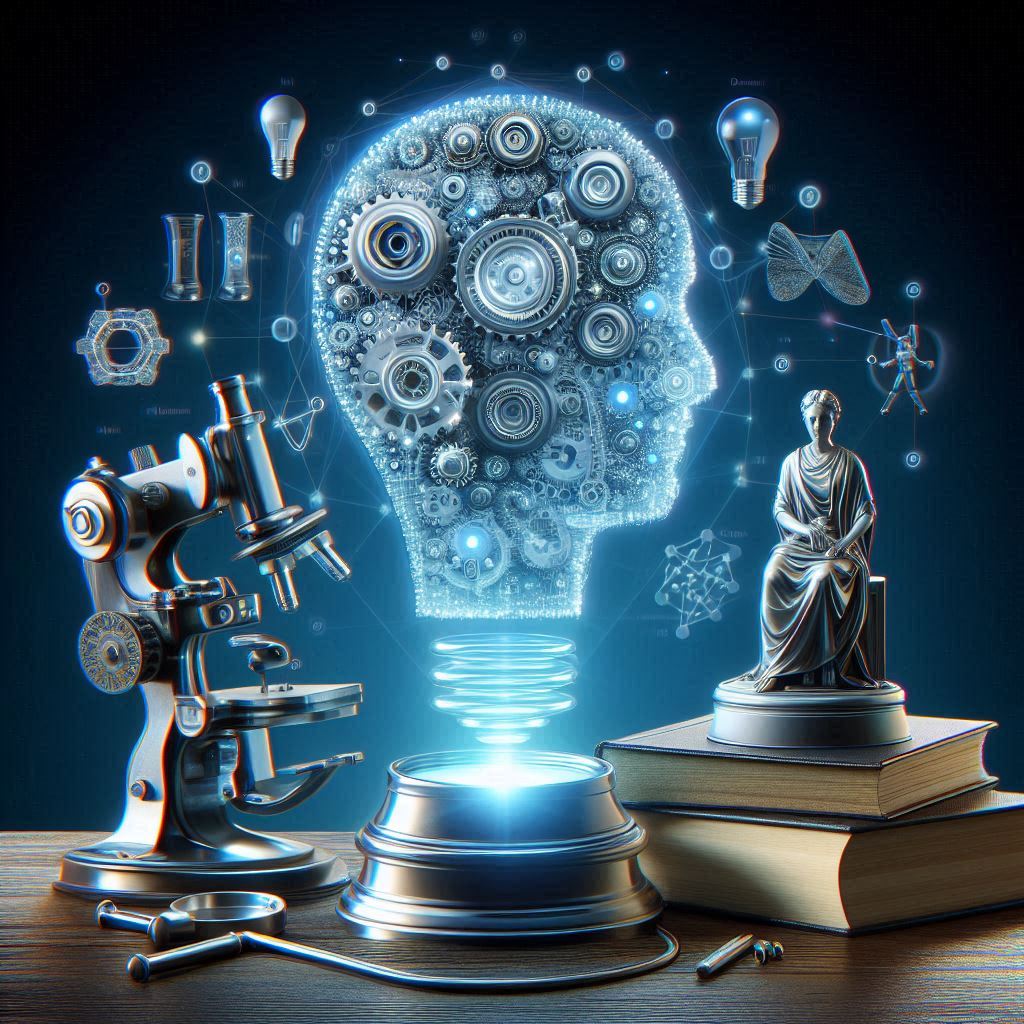
Pros and Cons of Machine Learning
Introduction
Machine learning (ML) has become a transformative technology across various sectors, enabling innovations and improvements in efficiency. However, like any technology, it has its advantages and challenges. This outline provides a comprehensive look at the pros and cons of machine learning.
Pros of Machine Learning
1. Enhanced Automation and Efficiency
Machine learning algorithms can automate complex tasks, leading to significant improvements in efficiency. For instance, in manufacturing, ML can optimize production lines and reduce downtime through predictive maintenance.
2. Improved Decision Making
ML models can analyze vast amounts of data and provide insights that drive better decision-making. This capability is valuable in various industries, including finance, healthcare, and marketing.
3. Personalization and Customer Experience
ML enables personalized experiences by analyzing user behavior and preferences. This personalization enhances customer satisfaction and engagement.
4. Scalability and Adaptability
ML systems can scale and adapt to new data, making them flexible solutions for a wide range of applications. This adaptability allows businesses to handle large datasets and evolving requirements.
Cons of Machine Learning
1. Data Privacy and Security Concerns
Machine learning often relies on large volumes of data, raising concerns about data privacy and security. The collection and use of personal data can lead to potential breaches and misuse.
2. Bias and Fairness Issues
Machine learning models can inadvertently perpetuate or even amplify biases present in the training data, leading to unfair or discriminatory outcomes.
3. Lack of Interpretability and Transparency
Many ML models, particularly deep learning systems, operate as “black boxes,” making it difficult to understand how they arrive at their conclusions. This lack of transparency can hinder trust and accountability.
4. High Costs and Resource Requirements
Developing and deploying machine learning models can be resource-intensive, requiring significant computational power and expertise. This can be a barrier for smaller organizations and startups.
Frequently Asked Questions (FAQs) About Machine Learning
1. What is Machine Learning?
Machine learning (ML) is a branch of artificial intelligence (AI) that focuses on building systems capable of learning from data and improving their performance over time without being explicitly programmed. ML uses algorithms to analyze data, identify patterns, and make predictions or decisions.
For a deeper understanding of ML, you can read more about it on Expert Market Research and IBM’s guide on Machine Learning.
2. What are the Different Types of Machine Learning?
Machine learning can be broadly categorized into several types:
- Supervised Learning: Involves training a model on labeled data, where the output is known. Common algorithms include linear regression and support vector machines (SVMs).
- Unsupervised Learning: Involves training a model on unlabeled data to uncover hidden patterns or structures. Techniques include K-means clustering and principal component analysis (PCA).
- Reinforcement Learning: Focuses on training models to make sequences of decisions by rewarding desired behaviors and punishing undesired ones. It’s used in robotics and game playing.
Explore further on Coursera’s overview of machine learning types.
3. What Are Some Common Applications of Machine Learning?
Machine learning has numerous applications across different industries:
- Healthcare: ML is used for predictive analytics, disease diagnosis, and personalized medicine.
- Finance: Applications include fraud detection, algorithmic trading, and risk management.
- Retail: ML enhances personalization, inventory management, and customer service.
- Transportation: ML is crucial for autonomous vehicles and route optimization.
4. How Does Machine Learning Differ from Artificial Intelligence?
Artificial Intelligence (AI) is a broader field that encompasses various technologies designed to mimic human intelligence. Machine learning is a subset of AI focused specifically on developing systems that can learn from data and improve over time. Essentially, all machine learning is AI, but not all AI is machine learning.
For a detailed comparison, visit TechRadar’s comparison of AI and Machine Learning.
5. What Are the Main Challenges Associated with Machine Learning?
Machine learning, while powerful, faces several challenges:
- Data Quality: The effectiveness of ML models depends on the quality of the data used. Poor data quality can lead to inaccurate predictions. Learn more about data quality challenges.
- Bias and Fairness: ML models can inherit biases from their training data, leading to discriminatory outcomes. Addressing these biases is critical to ensuring fairness.
- Interpretability: Many ML models, especially deep learning models, are often considered “black boxes” due to their complex nature. This makes it difficult to interpret their decision-making process.
- Resource Requirements: Training and deploying ML models can be resource-intensive, requiring substantial computational power and energy. Explore the economic impact of ML.
6. How Can I Get Started with Machine Learning?
To start learning machine learning, follow these steps:
- Understand the Basics: Gain foundational knowledge in statistics, programming, and linear algebra. Resources such as Khan Academy and Coursera offer introductory courses.
- Take Online Courses: Enroll in machine learning courses from platforms like Udacity and edX.
- Work on Projects: Apply your skills through practical projects. Websites like Kaggle provide datasets and competitions to work on.
- Join Communities: Engage with ML communities and forums such as Reddit’s r/MachineLearning or Certainly! Here’s a detailed disclaimer and caution for a document or article related to machine learning. This section is designed to provide a comprehensive overview of potential issues and limitations, ensuring that readers are well-informed about the content and its applications.
Disclaimer and Caution
1. General Disclaimer
The information provided in this document is intended for educational and informational purposes only. While efforts have been made to ensure the accuracy and completeness of the content, no guarantee is made that the information is free from errors or omissions. The content is based on the best available knowledge and practices at the time of writing, but advancements in technology, changes in industry standards, and other factors may render some of the information outdated or inaccurate.
Readers are advised to consult with a qualified professional or expert in the relevant field before making decisions based on the information provided. The authors and publishers of this document assume no responsibility for any actions taken or decisions made based on the content herein. For up-to-date information and advice, please refer to authoritative sources and professional guidance.
2. Accuracy and Completeness
Although this document aims to provide accurate and current information, it may not encompass all aspects of machine learning, including the latest developments and research. The field of machine learning is rapidly evolving, and new techniques, algorithms, and best practices are continually emerging. As such, some information may become obsolete or superseded by more recent advancements.
Readers should verify the accuracy of the information and consider consulting additional resources and peer-reviewed literature to obtain a comprehensive understanding. For updated information and resources, consider exploring reputable platforms such as arXiv for research papers and Medium’s machine learning section for industry insights.
3. No Professional Advice
The content provided does not constitute professional advice, including but not limited to legal, medical, or financial advice. Machine learning applications and implementations can vary widely based on context, industry, and specific requirements. Readers should seek professional consultation tailored to their individual needs and circumstances.
For specific professional advice, consult with qualified practitioners in the respective fields. For example, if machine learning is being applied in a healthcare setting, consulting with a healthcare IT professional or data scientist specializing in medical applications would be advisable.
4. Data Privacy and Security
Machine learning often involves the use of large datasets, which may include sensitive or personal information. The handling of such data must comply with relevant data protection regulations, such as the General Data Protection Regulation (GDPR) in the European Union or the California Consumer Privacy Act (CCPA) in the United States.
It is crucial to ensure that appropriate measures are in place to protect data privacy and security. This includes anonymizing personal data, implementing secure data storage solutions, and adhering to best practices for data encryption and access control. For further guidance on data privacy and security, refer to resources provided by organizations such as EU GDPR and PrivacyTools.io.
5. Ethical Considerations
Ethical considerations are paramount in the development and deployment of machine learning systems. Issues such as bias, fairness, and transparency must be addressed to ensure that ML models do not perpetuate discrimination or harm marginalized groups. The decisions made during the design and implementation of ML systems can have significant societal impacts.
It is essential to adopt ethical practices, including conducting regular audits for bias, ensuring fairness in algorithmic outcomes, and maintaining transparency about how ML models make decisions. For more information on ethical AI and machine learning, visit Ethics.org and MIT Technology Review’s guidelines on ethical AI.
6. Limitations of Machine Learning Models
Machine learning models have inherent limitations and may not always perform as expected in every scenario. These limitations can arise from factors such as the quality of the training data, the complexity of the model, and the assumptions made during the modeling process. Models may also struggle with generalization to new or unseen data, leading to performance issues.
It is important to understand the limitations of the models used and to continuously evaluate their performance in real-world applications. For further reading on model limitations and performance evaluation, refer to Towards Data Science’s guide on model evaluation and Analytics Vidhya’s interpretation guide.
7. Legal and Regulatory Compliance
The use of machine learning is subject to various legal and regulatory requirements, which may differ by jurisdiction. Compliance with these regulations is essential to avoid legal repercussions and to ensure ethical use of technology. Regulations may pertain to data protection, intellectual property, and industry-specific standards.
It is crucial to stay informed about relevant legal and regulatory frameworks and to consult with legal professionals when developing or deploying machine learning systems. For information on legal considerations in ML, visit Lawfare’s analysis on legal issues and CIO’s overview of data privacy laws.
8. Disclaimer on External Links
This document contains links to external websites for additional information and resources. These links are provided for convenience and do not constitute an endorsement of the content or the organizations behind them. The authors and publishers of this document are not responsible for the content or accuracy of external websites.
Readers are encouraged to evaluate the reliability and relevance of external sources and to consult multiple references when seeking information. For additional resources, consider visiting Google or Bing for further searches.
9. Acknowledgment of Source Material
The content of this document has been compiled from various sources and references. While every effort has been made to accurately represent the information, there may be discrepancies or updates that are not reflected. Readers are encouraged to consult primary sources and authoritative publications for the most accurate and current information.
For a thorough understanding, visit primary sources such as Springer’s books on ML and Elsevier’s journals on AI and ML.
10. Contact Information
If you have any questions or concerns regarding the content of this document or its applications, please contact the authors or the organization responsible for its publication. For general inquiries, you may also reach out to professional forums or communities related to machine learning and AI.
For further assistance, you can explore LinkedIn for professional connections and Reddit’s r/MachineLearning for community support.