Transforming Industries: The Impact of Machine Learning in 2024
Machine learning (ML) continues to revolutionize various industries, unlocking unprecedented capabilities and driving significant advancements. In 2024, its impact is more pronounced than ever, with machine learning applications permeating diverse sectors such as healthcare, finance, agriculture, and entertainment. This article explores how machine learning is transforming industries, highlighting success stories, exemplary cases, and the broader implications for the future.
Healthcare: Enhancing Diagnosis and Treatment
In healthcare, machine learning algorithms are enhancing diagnostic accuracy and treatment efficacy. For instance, ML models are now capable of analyzing medical images with remarkable precision, aiding in the early detection of diseases such as cancer. Companies like IBM Watson Health and Google Health are at the forefront of integrating ML into healthcare systems, providing tools that support doctors in making informed decisions.
Company | Application | Impact |
---|---|---|
IBM Watson Health | Medical Image Analysis | Improved diagnostic accuracy |
Google Health | Predictive Analytics | Early disease detection |
Finance: Revolutionizing Risk Assessment and Fraud Detection
The finance industry is leveraging machine learning to revolutionize risk assessment and fraud detection. ML algorithms can analyze vast datasets to identify patterns and anomalies that signify potential risks or fraudulent activities. Companies like SAS and Palantir are using these technologies to create more secure and efficient financial systems.
Company | Application | Impact |
---|---|---|
SAS | Fraud Detection | Reduced fraudulent transactions |
Palantir | Risk Assessment | Enhanced risk management |
Agriculture: Driving Precision Farming
In agriculture, machine learning is driving the adoption of precision farming techniques. These technologies enable farmers to optimize crop yields, reduce waste, and minimize environmental impact. Companies like John Deere and Bayer are leading the charge, offering ML-powered solutions that provide insights into soil health, weather patterns, and crop performance.
Company | Application | Impact |
---|---|---|
John Deere | Precision Farming | Optimized crop yields |
Bayer | Digital Farming | Reduced environmental impact |
Entertainment: Innovating Content Creation
The entertainment industry is harnessing machine learning to innovate content creation and enhance user experiences. From generating realistic visual effects to personalizing content recommendations, ML is transforming how content is produced and consumed. Companies like Netflix and Disney Research are leveraging ML to create immersive experiences that captivate audiences.
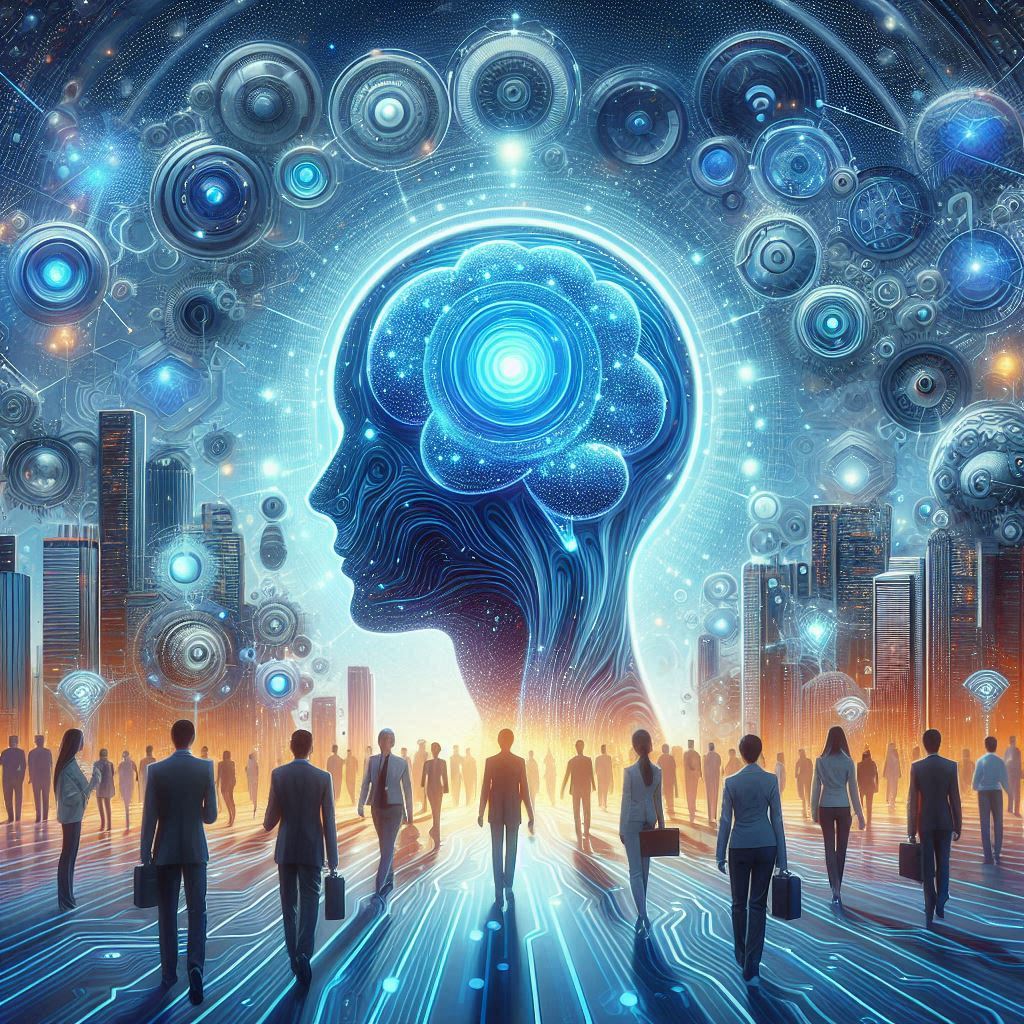
Company | Application | Impact |
---|---|---|
Netflix | Content Recommendation | Personalized user experiences |
Transforming Industries: The Impact of Machine Learning in 2024Machine learning (ML) continues to revolutionize various industries, unlocking unprecedented capabilities and driving significant advancements. In 2024, its impact is more pronounced than ever, with machine learning applications permeating diverse sectors such as healthcare, finance, agriculture, and entertainment. This article explores how machine learning is transforming industries, highlighting success stories, exemplary cases, and the broader implications for the future. |
Pros and Cons of Machine Learning in 2024
Pros
1. Enhanced Efficiency and Productivity:
Machine learning algorithms can process vast amounts of data quickly and accurately, significantly enhancing efficiency and productivity in various sectors. For instance, in manufacturing, ML can optimize production lines and predict maintenance needs, reducing downtime and costs.
2. Improved Decision Making:
By analyzing large datasets and identifying patterns, ML provides valuable insights that inform better decision-making. In finance, for example, ML models can predict market trends, assess credit risk, and detect fraudulent activities, leading to more informed and accurate financial decisions.
3. Personalization:
ML enables highly personalized experiences across various industries. In entertainment, streaming services like Netflix use ML algorithms to recommend content based on users’ viewing habits, enhancing user satisfaction and engagement.
4. Innovation and New Opportunities:
ML fosters innovation by enabling the development of new products and services. For instance, in healthcare, ML-driven diagnostic tools and personalized treatment plans are improving patient outcomes and creating new opportunities for medical research.
5. Predictive Capabilities:
ML’s predictive capabilities are transforming various industries. In agriculture, ML models can predict crop yields and disease outbreaks, helping farmers make proactive decisions to optimize their operations and mitigate risks.
Cons
1. Data Privacy and Security Concerns:
The extensive use of data in ML applications raises significant privacy and security concerns. Personal data used to train ML models can be vulnerable to breaches and misuse, leading to potential violations of privacy and data protection regulations.
2. Bias and Fairness Issues:
ML models can inadvertently perpetuate biases present in training data, leading to unfair and discriminatory outcomes. In finance, biased ML algorithms used for credit scoring can result in unfair lending practices, disproportionately affecting certain groups.
3. High Implementation Costs:
Developing and deploying ML solutions can be costly, requiring significant investment in technology, infrastructure, and expertise. Small and medium-sized enterprises (SMEs) may struggle to afford these costs, limiting their ability to benefit from ML advancements.
4. Complexity and Lack of Understanding:
ML technologies can be complex and difficult to understand, creating barriers to adoption. A lack of understanding about how ML models work can lead to mistrust and resistance from both organizations and the public.
5. Dependency on Quality Data:
The accuracy and effectiveness of ML models depend heavily on the quality of the data used for training. Poor-quality or biased data can result in unreliable models, reducing the overall benefits of ML applications.
Conclusion
The impact of machine learning in 2024 is profound, driving transformative changes across various industries. While the benefits of enhanced efficiency, improved decision-making, personalization, innovation, and predictive capabilities are substantial, it is crucial to address the challenges of data privacy, bias, implementation costs, complexity, and data quality. By navigating these pros and cons, industries can harness the full potential of machine learning to achieve significant advancements and create a more innovative and efficient future.
FAQs on Machine Learning’s Impact in 2024
Machine learning (ML) continues to transform various industries by offering innovative solutions and driving efficiency. Here, we address some frequently asked questions about ML’s impact in 2024.
What is machine learning?
Machine learning is a subset of artificial intelligence (AI) that involves the use of algorithms and statistical models to enable computers to learn from and make predictions or decisions based on data. Unlike traditional programming, where rules are explicitly coded, ML systems improve their performance as they are exposed to more data over time. For a detailed overview, visit IBM’s machine learning guide.
How is machine learning used in healthcare?
In healthcare, machine learning is used to enhance diagnostic accuracy, predict patient outcomes, and personalize treatment plans. For example, ML models can analyze medical images to detect diseases early or predict patient deterioration based on electronic health records. Companies like IBM Watson Health and Google Health are leading the integration of ML into healthcare systems. Learn more from Healthcare IT News.
What are the benefits of machine learning in finance?
Machine learning enhances finance by improving risk assessment, fraud detection, and investment strategies. ML algorithms can analyze large datasets to identify patterns and predict market trends, assess credit risk more accurately, and detect fraudulent activities. Companies like SAS and Palantir are at the forefront of these innovations. For more insights, visit Forbes.
How does machine learning benefit agriculture?
Machine learning benefits agriculture by enabling precision farming techniques. ML models can predict crop yields, monitor soil health, and identify pests or diseases, helping farmers make data-driven decisions. Companies like John Deere and Bayer provide ML-powered solutions to optimize farming practices. Discover more from AgFunderNews.
What are the challenges of implementing machine learning?
Implementing machine learning comes with several challenges, including data privacy and security concerns, bias in algorithms, high implementation costs, and the need for quality data. Ensuring that ML models are transparent and fair is crucial to gaining public trust and avoiding unintended consequences. For a deeper understanding, refer to Brookings Institution.
How is machine learning transforming the entertainment industry?
Machine learning is transforming the entertainment industry by personalizing content recommendations, enhancing visual effects, and optimizing production processes. Streaming services like Netflix use ML to recommend shows and movies based on user preferences, while companies like Disney Research leverage ML to create realistic animations and visual effects. For more information, check out MIT Technology Review.
What is the future of machine learning?
The future of machine learning is promising, with continued advancements expected to further revolutionize various industries. Innovations in natural language processing, reinforcement learning, and federated learning will enable even more sophisticated applications. As ML technologies evolve, ethical considerations and regulatory frameworks will play a crucial role in shaping their development. For a comprehensive outlook, visit Gartner.
How can businesses get started with machine learning?
Businesses can get started with machine learning by identifying specific use cases where ML can add value, such as improving customer service, optimizing supply chains, or enhancing product recommendations. It’s essential to have a clear strategy, invest in the right technology and expertise, and ensure access to quality data. Collaborating with experienced ML solution providers can also facilitate a smoother implementation process. For practical steps, refer to Harvard Business Review.
What are some real-world examples of machine learning success stories?
Real-world examples of machine learning success stories span various industries. In healthcare, IBM Watson Health has developed ML-driven tools for early disease detection. In finance, Ant Financial uses ML for efficient fraud detection. In agriculture, Corteva Agriscience leverages ML to improve crop management. For more examples, explore Forbes.
Disclaimer and Caution Regarding Machine Learning in 2024
As machine learning (ML) continues to advance and integrate into various sectors, it is essential to understand both its potential and its limitations. This disclaimer and cautionary note aim to provide a comprehensive overview of the considerations that should be kept in mind when implementing and relying on ML technologies in 2024.
General Disclaimer
The information provided in this article is intended for general informational purposes only. While we strive to ensure the accuracy and reliability of the information presented, we do not make any warranties or guarantees, express or implied, regarding the completeness, accuracy, reliability, suitability, or availability of the content. Any reliance you place on such information is strictly at your own risk.
Limitations of Machine Learning
Machine learning is a powerful tool, but it has its limitations. ML models are only as good as the data they are trained on. Poor quality, biased, or incomplete data can lead to inaccurate or unfair results. Additionally, ML models can be complex and difficult to interpret, making it challenging to understand how decisions are made. It is crucial to consider these limitations when implementing ML solutions.
Ethical and Legal Considerations
The use of machine learning raises important ethical and legal questions. Issues such as data privacy, consent, and bias must be addressed to ensure that ML applications are fair and ethical. Organizations must comply with relevant laws and regulations, including data protection and anti-discrimination laws. Failure to do so can result in legal consequences and damage to reputation.
For more information on ethical considerations, visit Brookings Institution.
Security Risks
Machine learning systems can be vulnerable to security threats, including data breaches and adversarial attacks. Adversarial attacks involve manipulating input data to deceive ML models, leading to incorrect outputs. Organizations must implement robust security measures to protect ML systems and the data they process. Regular security audits and updates are essential to mitigate these risks.
Learn more about ML security from CSO Online.
Responsibility and Accountability
The deployment of machine learning systems requires clear accountability and responsibility frameworks. Organizations must designate individuals or teams responsible for overseeing ML projects, ensuring ethical use, and addressing any issues that arise. Transparency in ML processes and decision-making is critical to maintaining trust and accountability.
Bias and Fairness
Bias in ML models can lead to unfair outcomes and reinforce existing inequalities. It is crucial to identify and mitigate bias during the development and deployment of ML systems. Techniques such as diverse data collection, bias testing, and fairness-aware algorithms can help reduce bias. However, achieving fairness in ML is an ongoing challenge that requires continuous effort and vigilance.
For further reading, refer to FairMLBook.
Quality of Data
The effectiveness of machine learning models heavily depends on the quality of the data used for training. High-quality, representative, and unbiased data is essential for accurate and reliable ML models. Organizations must invest in data quality management practices, including data cleaning, validation, and enrichment, to ensure the success of ML applications.
Complexity and Interpretability
Machine learning models, especially deep learning models, can be complex and difficult to interpret. This “black box” nature makes it challenging to understand how decisions are made, which can be problematic in critical applications such as healthcare and finance. Efforts to improve interpretability, such as explainable AI (XAI) techniques, are essential to ensure transparency and trust in ML systems.
High Implementation Costs
Implementing machine learning solutions can be expensive, requiring significant investment in technology, infrastructure, and expertise. Small and medium-sized enterprises (SMEs) may find it challenging to afford these costs, limiting their ability to benefit from ML advancements. Organizations must carefully assess the return on investment (ROI) and consider cost-effective alternatives when planning ML projects.
Regulatory Compliance
Organizations must ensure that their use of machine learning complies with relevant regulations and standards. This includes data protection laws, such as the General Data Protection Regulation (GDPR), and industry-specific regulations. Non-compliance can result in legal penalties and reputational damage. Staying informed about regulatory changes and implementing compliance frameworks is crucial.
For more on regulatory compliance, see GDPR.eu.
Ongoing Maintenance and Monitoring
Machine learning models require ongoing maintenance and monitoring to ensure they continue to perform effectively. Changes in data patterns, user behavior, and external factors can impact model performance. Regular updates, retraining, and performance monitoring are essential to maintain the accuracy and reliability of ML systems.
For more details, visit KDnuggets.
Conclusion
While machine learning offers significant benefits and transformative potential, it is crucial to approach its implementation with caution. Understanding the limitations, ethical considerations, security risks, and regulatory requirements is essential to ensure the responsible and effective use of ML technologies. By addressing these challenges, organizations can harness the power of machine learning to drive innovation and create value while maintaining trust and accountability.